Revolutionizing Railways: AI-Powered Insights for Modern Transportation Efficiency
Total images | : 4,849 |
Type | : organic |
Category | : Objects |
Resolution | : Up to 1024px |
Storage size | : Up to 1017 Mb |
File format | : JPEG |
The Railway Transport Collection dataset is a comprehensive and meticulously collected compilation of diverse information related to all aspects of rail transport. This dataset serves as a valuable resource for researchers, analysts, and enthusiasts interested in gaining insights into the world of railways. It encompasses a wide range of data, including historical records, infrastructure details, rolling stock specifications, operational statistics, safety measures, and technological advancements within the realm of rail transportation.
Whether you are a transportation professional seeking to enhance operational strategies, an urban planner looking to integrate efficient transit solutions, or simply an individual passionate about trains and railways, the Railway Transport Collection dataset is an invaluable repository of knowledge that promises to enrich your understanding of this vital mode of transportation.
The Railway Transport Collection dataset offers a wealth of data that can be leveraged for various AI and machine learning use cases, contributing to advancements in the field of rail transport.
1. Route Optimization: Machine learning algorithms can analyze various factors, such as distance, terrain, and speed limits, to optimize freight train routes, reducing travel time and fuel consumption
2. Traffic Forecasting: AI models can analyze historical train schedules, passenger data, and external factors like events and weather to predict future passenger and freight traffic patterns. This aids in optimizing resources, managing capacity, and ensuring smooth operations.
3. Anomaly Detection: Machine learning algorithms can monitor real-time data streams from railway systems to identify anomalies or unusual patterns that might indicate safety issues, track damage, or potential disruptions. This early detection helps prevent accidents and minimize service interruptions.
4. Optimized Scheduling: AI algorithms can optimize train schedules by considering factors like station congestion, maintenance windows, and track availability. This results in improved efficiency, reduced delays, and better utilization of resources.
5. Energy Efficiency: Machine learning can analyze data on train speeds, routes, and energy consumption to optimize energy usage. This helps in reducing carbon emissions, minimizing operational costs, and making rail transport more environmentally friendly.
6. Demand Forecasting: By examining historical passenger data and external factors, AI models can predict peak travel times, enabling better allocation of resources and ensuring passenger comfort during high-demand periods.
Environment: Commercial stock
Angle: Random
Augmentation: None
AR: Various
ACCURACY
This dataset contains a tolerance margin of 5% to 10% of associated images which might not reflect 100% accuracy in the metadata or image. As example for the error margin: a close up of a locomotive can appear, due to its association with trains and railway infrastructure. All metadata in this dataset had been created manually and might contain a low margin of error. The maximum resolution of each image might vary.
128px
$69
256px
$199
512px
$249
1024px
$349
Sample images in this dataset
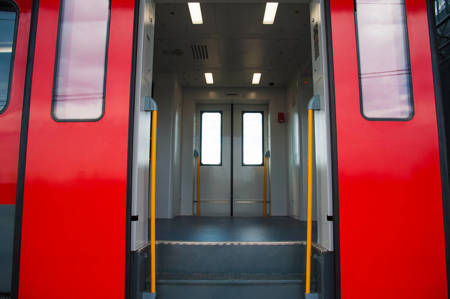
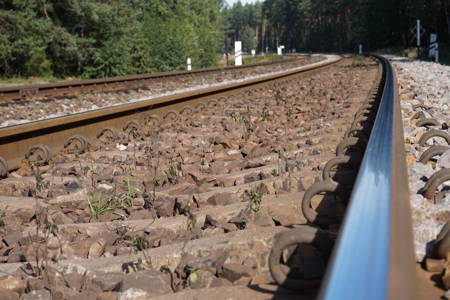
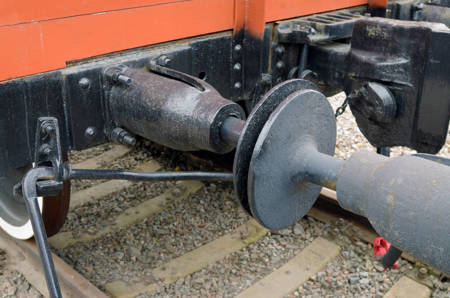
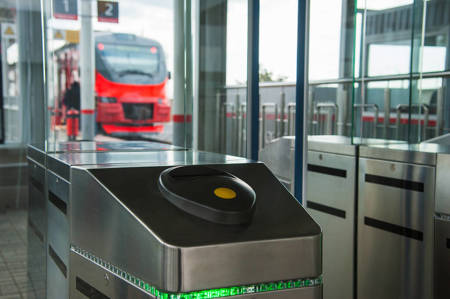
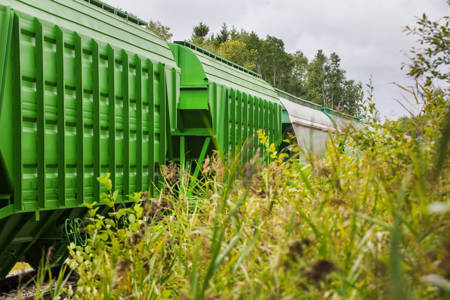
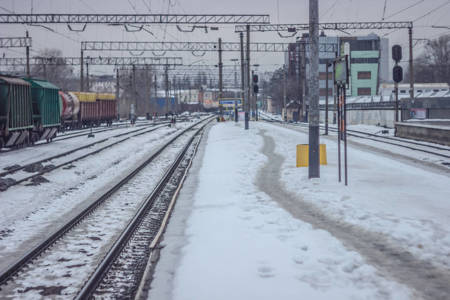
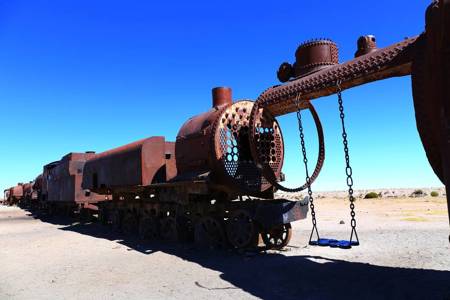
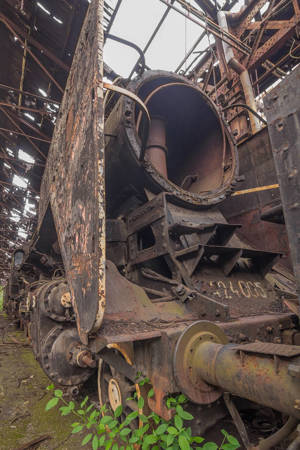
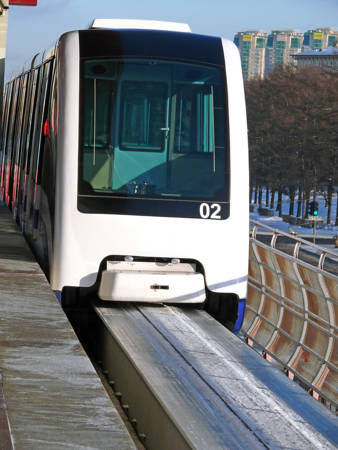
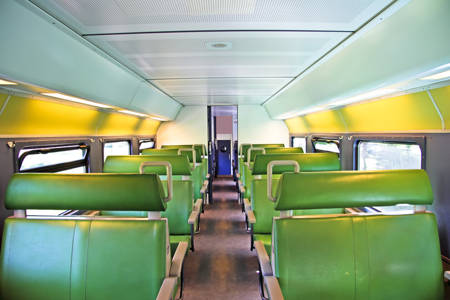
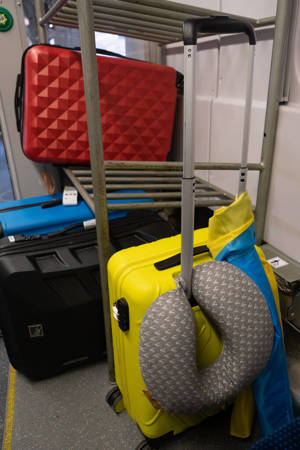
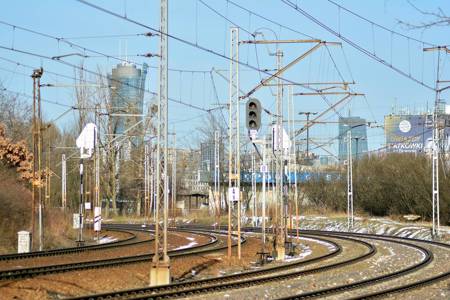
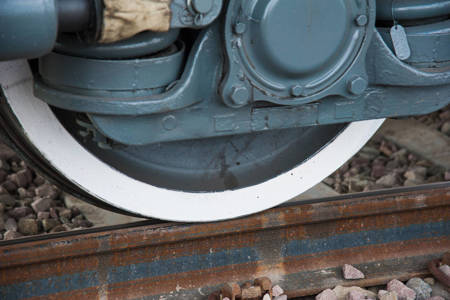
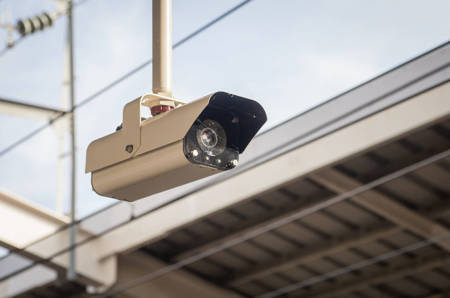
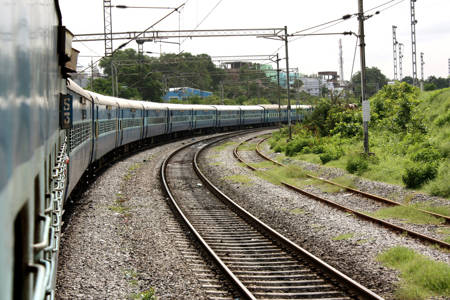
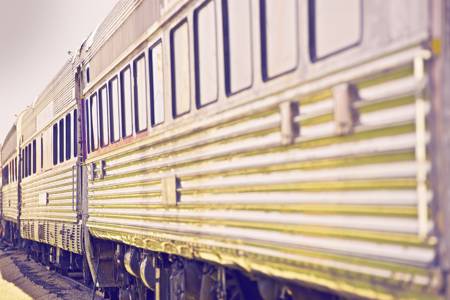
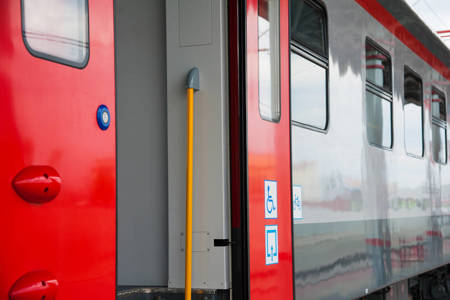
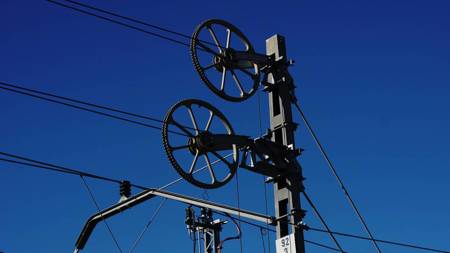
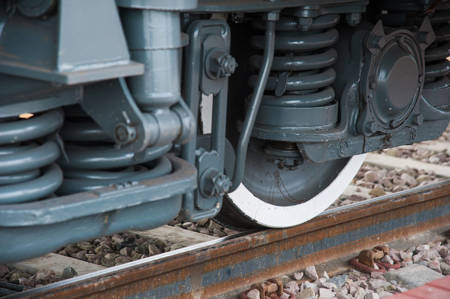
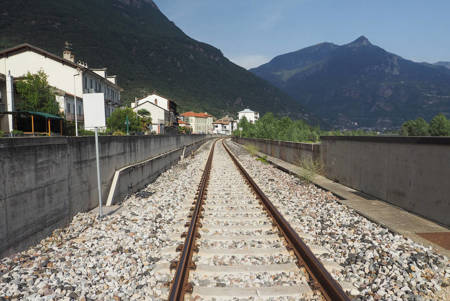
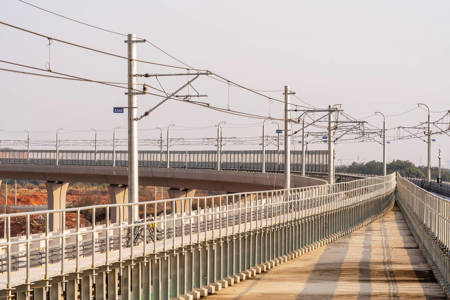
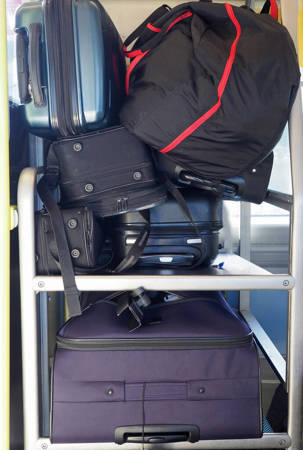
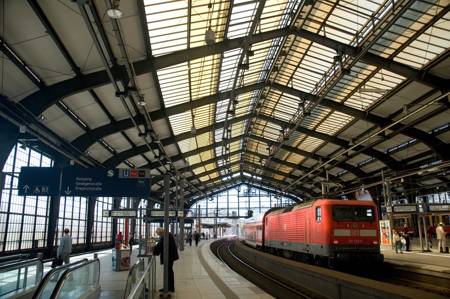
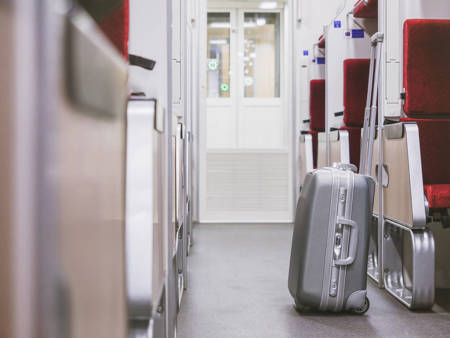
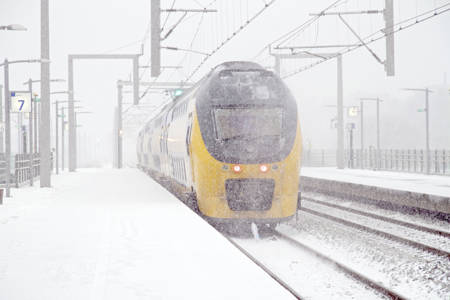
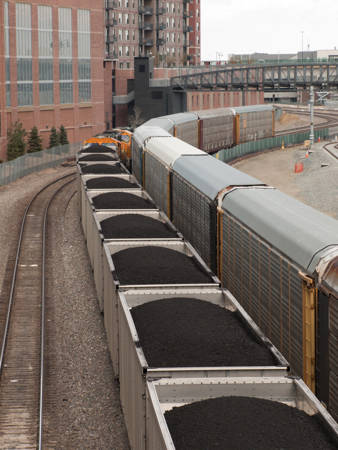
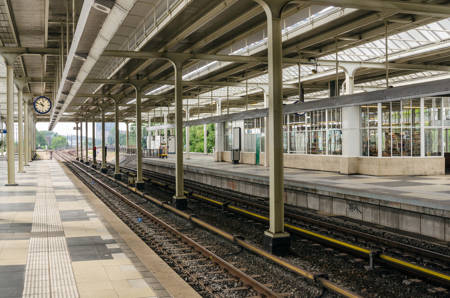
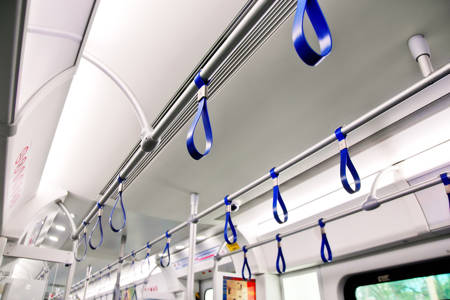
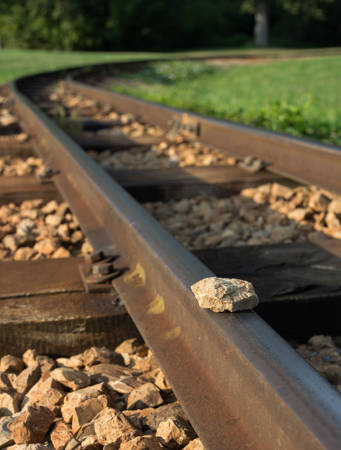
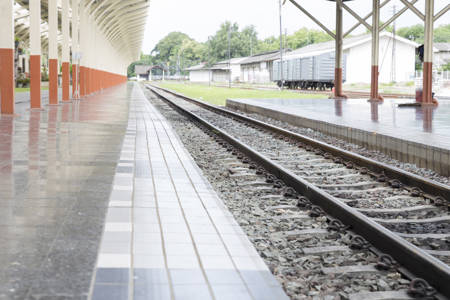
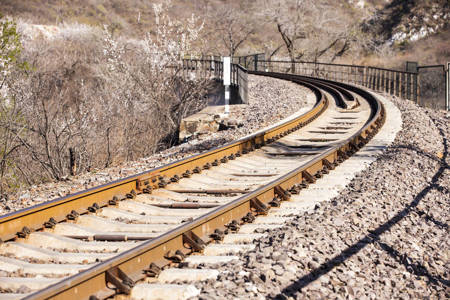
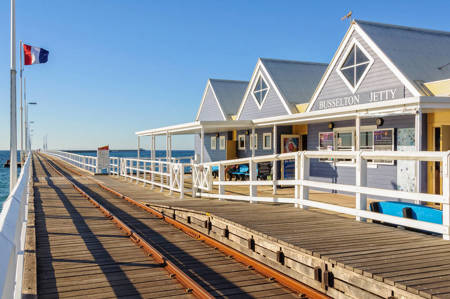
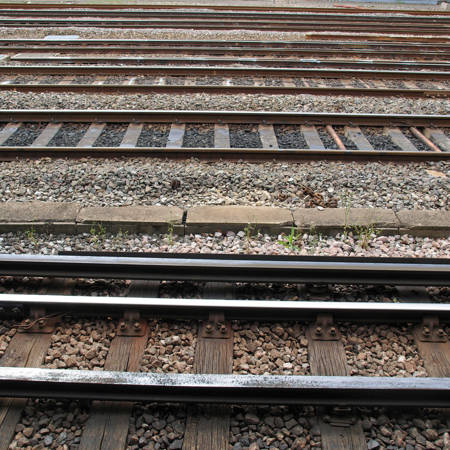
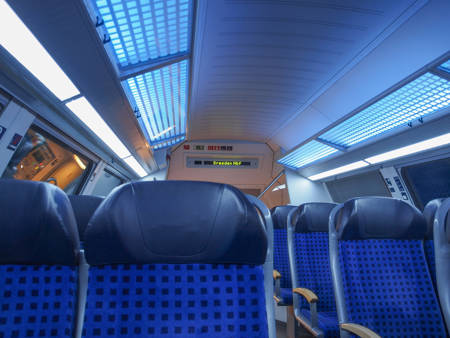
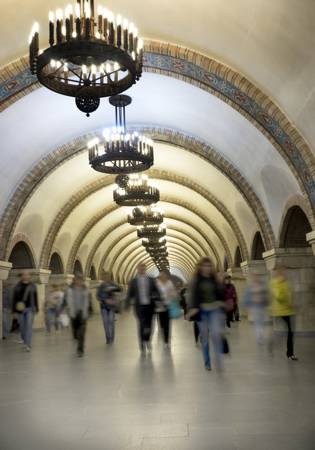
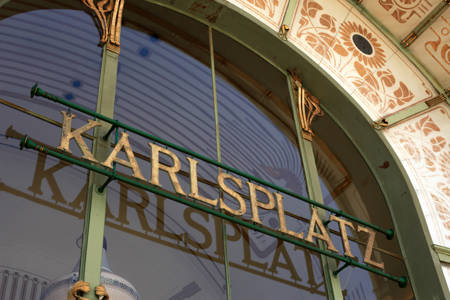
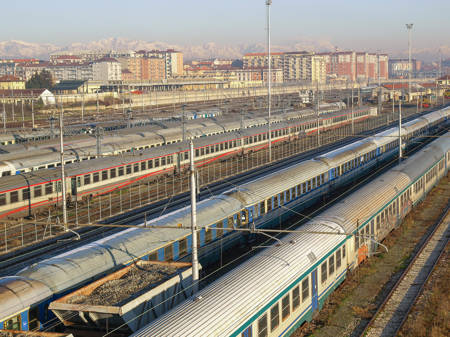
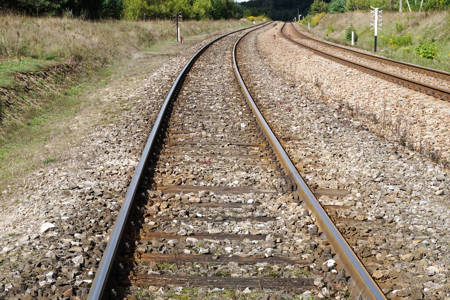
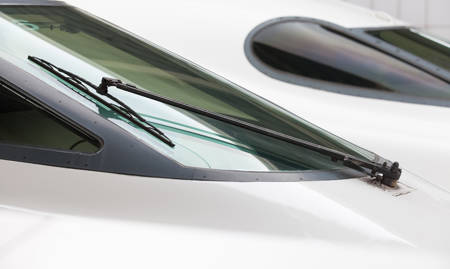
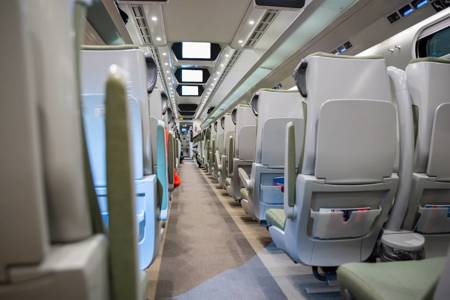
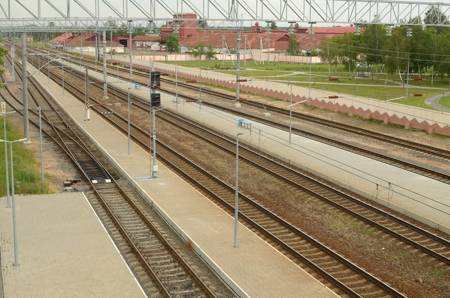
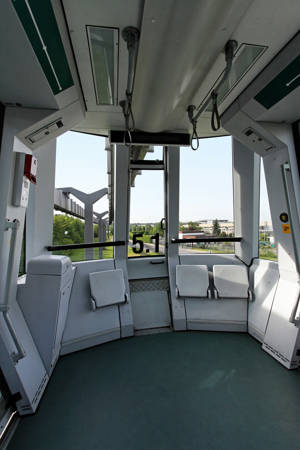
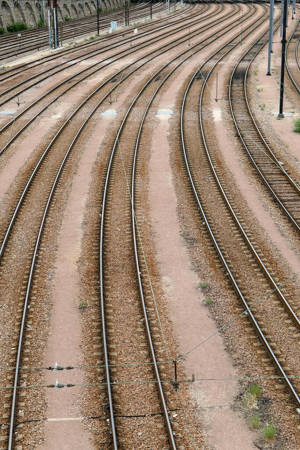
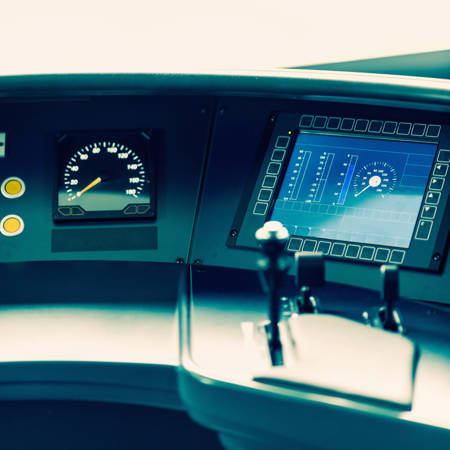
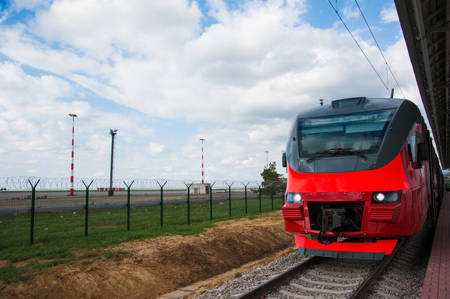